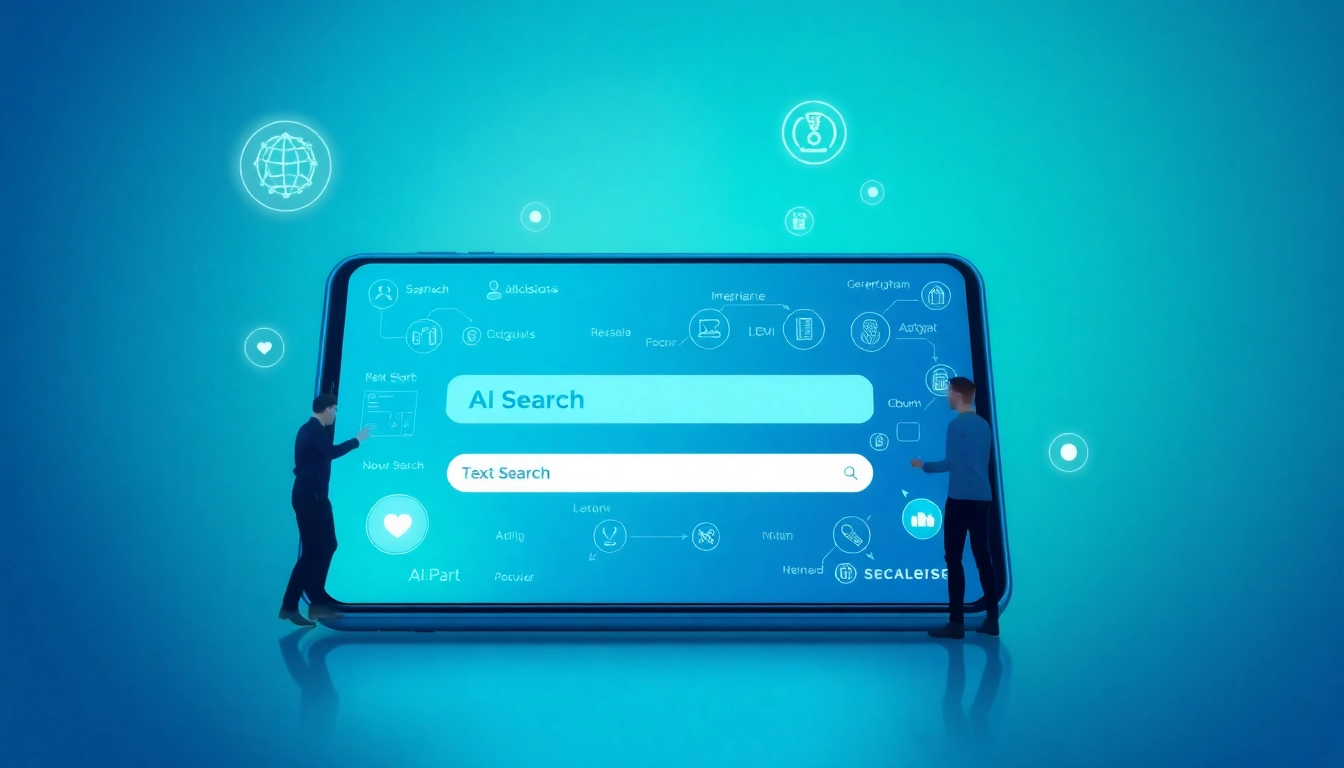
Understanding AI Search Technology
Artificial intelligence has revolutionized numerous industries, and the realm of search technology is no exception. AI search encompasses a variety of systems and technologies designed to enhance the information retrieval process. With the increasing volume of data generated every second, AI search systems are becoming indispensable tools for both individuals and organizations in navigating this information overload.
What is AI Search?
AI search refers to the application of artificial intelligence techniques to improve search capabilities. Unlike traditional search engines that rely solely on keyword matching and page rank, AI-driven search engines utilize deep learning algorithms, natural language processing (NLP), and machine learning to understand the intent behind user queries. This allows for more accurate and relevant results.
The emergence of AI search engines is driven by the need for enhanced search capabilities that can go beyond simple text matching. They are designed to interpret user intent, enhance semantic understanding, and provide contextually relevant information. Popular models include Google’s Gemini and OpenAI’s ChatGPT, both of which harness AI to deliver personalized and insightful responses to user inquiries.
How AI Search Works
AI search systems incorporate multiple technologies that work in synergy to improve the search experience:
- Natural Language Processing (NLP): This involves breaking down the user’s query into understandable language, translating it into a format that the machine can analyze.
- Machine Learning (ML): These algorithms learn from user interactions and feedback to improve the accuracy of future search results over time.
- Deep Learning: Utilized for recognizing patterns within large datasets, this allows for the processing of complex queries and nuanced content.
- Semantic Search: This technology focuses on the searcher’s intent and the contextual meaning of terms rather than just matching keywords.
By leveraging these technologies, AI search engines can generate results that are not only relevant but also contextually meaningful, thus navigating the challenges posed by ambiguity and the extensive variety of data available.
Types of AI Search Systems
AI search systems can be categorized into several types, each serving distinct purposes:
- Conversational Search Engines: These tools, like those powered by OpenAI, enable users to interact through natural conversation, asking follow-up questions and getting answers in a dialogue format.
- Visual Search Engines: By using image recognition technologies, these search engines allow users to conduct searches based on images rather than text, such as Google Lens.
- Enterprise Search Solutions: Solutions such as Azure AI Search focus on organizational needs, allowing employees to retrieve information from complex databases efficiently.
- Vertical Search Engines: These are specialized AI search engines that cater to specific niches or industries, providing tailored search results based on more specific data sets.
Advantages of AI Search
Increased Efficiency in Information Retrieval
One of the primary advantages of AI search is the significant increase in efficiency. Traditional search engines often return a large number of results, many of which may be irrelevant or require additional refinement of search terms to yield useful information. AI search reduces this burden by understanding user intent, allowing for more targeted results.
This efficiency translates into time savings for users, enabling them to find the information they need faster and with fewer repetitive queries. For instance, enterprise applications equipped with AI search can sift through vast collections of company data in mere seconds, significantly improving productivity.
Enhanced User Experience and Interaction
AI search engines offer a more interactive and engaging experience for users. The conversational interfaces allow users to engage dynamically, often benefiting from follow-up questions and clarifications that lead to more informative answers. This experience creates a sense of interaction akin to having a conversation with a knowledgeable assistant, thus enhancing user satisfaction.
The personalized nature of AI search also contributes to enriching user experience. By learning from individual user behaviors and preferences, these systems can curate results that align better with users’ needs and expectations.
Real-world Applications of AI Search
AI search technology is finding applications across various sectors:
- Healthcare: AI search is utilized for rapidly retrieving medical information, research papers, and patient data management, assisting healthcare professionals in making informed decisions.
- E-commerce: By analyzing consumer behavior, AI search allows retailers to provide personalized recommendations, thereby enhancing customer satisfaction and increasing sales.
- Finance: Financial institutions employ AI search to analyze vast datasets, aiding in market research, fraud detection, and customer service optimization.
- Education: In e-learning platforms, AI search helps students find relevant resources and tailor learning experiences, improving educational outcomes.
Challenges Facing AI Search Technology
Data Privacy and Security Concerns
The increasing reliance on AI search raises significant data privacy and security concerns. As users engage with AI systems, they often share personal information, which can be vulnerable to breaches. Additionally, the data collected for training AI models may inadvertently lead to exploitation or misuse if not handled appropriately.
To combat these concerns, companies must implement robust data governance frameworks, ensuring transparency around data use and prioritizing user consent. Encryption technologies and anonymization techniques can also play a critical role in protecting user data while still allowing for effective machine learning.
Accuracy and Reliability of AI Search Results
While AI search engines have made great strides in delivering relevant results, they still face challenges regarding the accuracy and reliability of information. The training data used to develop these models may contain biases or inaccuracies, which can lead to deceptive results. Furthermore, not all sources of information employ rigorous standards of accuracy, potentially affecting the quality of output.
To minimize these issues, organizations need to adopt continuous evaluation and improvement techniques. Incorporating feedback loops where users can report inaccuracies can also help in fine-tuning AI search algorithms.
Overcoming Bias in AI Algorithms
AI systems, including search engines, can perpetuate and amplify existing biases present in the underlying training data. This can lead to skewed results, impacting user trust and overall effectiveness. Addressing algorithmic bias requires comprehensive approaches, focusing on more inclusive data collection, algorithm transparency, and the continuous auditing of results for fairness.
Engaging diverse teams in the development process and employing tools designed to detect bias will further enhance the fairness and inclusivity of AI search results, leading to improved user interactions and societal benefits.
Best Practices for Implementing AI Search
Selecting the Right AI Search Solution
Choosing the right AI search solution is vital for organizations looking to leverage this technology effectively. Considerations should include administrative requirements, the complexity of data, existing infrastructure compatibility, and user goals. It is essential to evaluate different options against these criteria and consider trial periods or demonstrations to assess fit.
Integrating AI Search into Existing Systems
Successful integration of AI search requires a methodical approach. Organizations should ensure that new systems can seamlessly interact with existing data repositories and user workflows. Developing a clear migration strategy to transition users from traditional search methods to AI-enhanced systems is paramount, accompanied by comprehensive training programs to ease this transition.
Evaluating Performance and User Feedback
Organizations should continuously assess the performance of their AI search systems based on user interactions and feedback. Key performance indicators (KPIs) such as accuracy of results, user satisfaction scores, and engagement rates are essential in evaluating effectiveness. Administering periodic surveys and utilizing analytics tools provides insights that can drive improvements in system design and functionality.
The Future of AI Search
Emerging Trends in AI Search
As AI search technology continues to evolve, several trends are expected to dominate the landscape:
- Integration of Multimodal Search: Future AI search systems will likely enhance their capabilities by incorporating multimodal search, allowing users to query data via text, speech, and images simultaneously.
- Contextual Awareness: Advancements in NLP will enable search engines to be more contextually aware, understanding the nuances of human language and delivering results that anticipate user needs.
- Real-time Adaptation: AI search engines will become increasingly capable of adapting in real time based on user behavior and external factors, optimizing search results instantaneously.
Predicted Innovations in AI Search Technologies
Artificial intelligence is set to transform search technologies further with predicted innovations:
- Personalized Search Experiences: Leveraging user data to create tailored search experiences will become standard, leading to deeper user engagement and satisfaction.
- Greater Transparency: As issues regarding algorithmic bias and data privacy persist, there will be an increased demand for transparency in AI models, allowing users to understand how results are generated.
- Collaborative Search: Future AI systems may enable collaborative search experiences, where multiple users can interact and share inputs in real-time, creating a more dynamic search environment.
Potential Impacts on Industries and Society
The growing prevalence of AI search technology is poised to have far-reaching impacts across industries and society at large. As organizations adopt more sophisticated search tools, there is potential for enhanced productivity, better decision-making, and increased access to information.
Moreover, AI search can democratize access to knowledge, empowering individuals to analyze and utilize information in ways previously unavailable. However, it also raises ethical considerations regarding data privacy, information accuracy, and the potential for misuse, necessitating thoughtful governance and oversight as this technology continues to develop.